Visual Abstract
Abstract
This study investigates the stability, reactivity, and antiviral potential of novel 1,3,4-thiadiazole and 1,3-thiazolidine-4-one derivatives using a combination of Density Functional Theory (DFT) calculations and molecular docking. DFT analyses, including molecular electrostatic potential (MEP) mapping, reactivity indices (electronegativity, electrophilic index, softness, and hardness), and frontier molecular orbitals (HOMO-LUMO), were conducted to understand the chemical properties of the main compound. PASS predictions indicated strong activity of these compounds as Mcl-1 antagonists and antivirals. The docking studies, performed using AutoDock Vina v1.1.2 in PyRx 8, evaluated the binding affinities of 21 compounds against SARS-CoV-2 and rhinovirus, comparing them with standard drugs (Lopinavir, Nafamostat, and Remdesivir). The compounds exhibited binding affinities ranging from -6.9 to -8.5 kcal/mol, suggesting notable antiviral activity. Additionally, ADMET analysis (absorption, distribution, metabolism, excretion, and toxicity) was carried out using ADMET-AI and admetSAR 2.0, confirming their drug-like properties and suitability for further medicinal chemistry development.
Introduction
Respiratory disorders, particularly those caused by the novel Severe Acute Respiratory Syndrome Coronavirus 2 (SARS-CoV–2), have emerged as major public health challenges due to the scarcity of reliable antiviral therapies [1,2]. Researchers have focused on repurposing existing drugs and employing in silico methods—computer-based simulations—to discover potential treatments. Among the promising candidates are 1,3,4-thiadiazole derivatives, which have shown potential for treating COVID–19 [3,4]. The 1,3,4-thiadiazole nucleus is a notable heterocyclic structure in numerous natural products and pharmaceuticals [5]. It plays a central role in various drug classes, including antimicrobial [6], anti-inflammatory [7], analgesic [8], antiepileptic [9], antiviral [10], antineoplastic [11], and antitubercular agents [12]. The broad spectrum of biological activities exhibited by thiadiazole and its derivatives has made them invaluable as pharmacological scaffolds.
Similarly, the 4-thiazolidinone ring system contains many biologically active compounds with demonstrated antibacterial [13], antitubercular, antifungal [14], antimalarial [15], and antiviral effects [10]. Incorporating these structural motifs into drug design may lead to the development of novel therapeutics for a range of diseases, including COVID–19. However, rigorous scientific research and clinical trials are essential to establish the efficacy and safety of these compounds before they can be considered viable treatment options. Proper medical oversight is crucial to mitigate potential risks and ensure patients receive the best possible care. Researchers have already synthesized 1,3,4-thiadiazole derivatives with anticancer properties. Systematic altering substituents on the thiadiazole ring can fine-tune these compounds’ pharmacokinetic and pharmacodynamic profiles, enhancing their potential as therapeutic agents. The dual action of 1,3,4-thiadiazole derivatives may provide a multifaceted approach to treating COVID–19 by addressing both the viral load and the hyper-inflammatory responses observed in severe cases. Currently, no specific antiviral treatment is highly effective for SARS‐CoV‐2. As a result, numerous studies are being conducted to explore the potential use of existing antiviral medications either alone or in combination, as well as to devise new therapeutic drugs aimed at effectively treating COVID‐19. As research progresses, collaborative efforts between computational chemistry, medicinal chemistry, and pharmacology will be critical. In silico docking studies, combined with experimental validation, will help elucidate the precise mechanisms by which these thiadiazole derivatives exert their antiviral effects. Strategic clinical trials must be designed to evaluate the efficacy, safety, and optimal dosing regimens of these new agents to accelerate the translation of these findings into clinical practice. Multidisciplinary collaboration will be key to advancing drug repurposing while maintaining the highest scientific rigor and ethical responsibility standards.
This study involved selecting 21 derivatives of 1,3,4-thiadiazole and 1,3-thiazolidine–4-one binary molecules from the literature [16]. The next steps involved conducting DFT analysis of compound (Z)–5-((Z)-Benzylidene)–2-((5-(benzylthio)–1,3,4-thiadiazole–2-yl)imino)thiazolidine–4-one (1a), predicting the PASS (Prediction of Activity Spectra for Substances) of selected ligands, performing molecular docking studies of these ligands and three FDA-approved antiviral drugs (Lopinavir, Nafamostat, and Remdesivir, as shown in Figure 1) against COVID–19. Additionally, the ADMET properties of the three ligands that docked in the same active site as the standard ligands were assessed, and their structures were optimized to improve bioavailability.
Materials and methods
Computational studies were performed using Gaussian 09 [17] and Gaussview 05. The optimized geometry and the geometrical parameters were computed using Density Functional Theory (DFT) with (B3LYP/6–311++G(d,p) basis set. Protein targets were downloaded from Protein (http://www.rcsb.org).
The PASS online (way2drug.com/passonline/predict.php) was used to predict the biological activities [18] ADMET-AI [19] is a user-friendly web interface with machine learning models used to predict the ADMET properties of molecules. AdmetSAR 3.0 server (http://lmmd.ecust.edu.cn/admetsar3/index.php) was used for ADMET optimization [20].
Results and Discussion
Structure optimization
The optimized structural parameters, including bond lengths and bond angles, of 1a were calculated using the DFT/B3LYP method with a 6–311++G(d,p) basis set in the gas phase. The results are presented in Table 1 (Figure 2). An excellent agreement was observed between the experimental [16] and theoretical results. The differences between the theoretical DFT results in the gas phase and the experimental results in the solid phase are minimal, mainly due to the phase difference. In the 1,3,4-thiadiazole structure, the bond lengths for C(17)-N(18), N(18)-N(19), C(15)-N(19), and S(16)-C(15) were calculated/observed as 1.304/1.303 Å, 1.366/1.383 Å, 1.304/1.301 Å, and 1.768/1.740 Å, respectively. The bond angles for C(15)-S(16)-C(17), C(15)-N(19)-N(18), C(17)-N(18)-N(19), and S(16)-C(17)-N(18) were calculated/observed as 85.844°/86.813°, 113.800°/112.612°, 113.006°/112.530°, and 114.093°/114.237°, respectively.
In the 1,3-thiazolidine–4-one structure, the bond lengths for C(8)-C(9), C(9)-N(10), N(10)-C(11), C(11)-S(12), and S(12)-C(8) were calculated/observed as 1.489/1.479 Å, 1.390/1.369 Å, 1.374/1.375 Å, 1.782/1.762 Å, and 1.782/1.769 Å, respectively. The bond angles for C(8)-C(9)-N(10), C(9)-N(10)-C(11), N(10)-C(11)-S(12), and S(12)-C(8)-C(9) were calculated/observed as 108.861°/110.292°, 118.997°/117.867°, 110.078°/110.348°, and 110.900°/109.897°, respectively. The bond angle for C(11)-N(14)-C(15) was calculated/observed as 121.821°/120.491°.
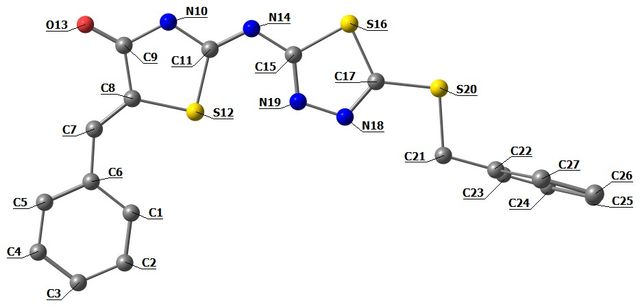
Bond length (bond order) | Calculated |
C(2)- C(1) | 1.390 |
C(3)- C(2) | 1.40 |
C(4)- C(3) | 1.390 |
C(5)- C(4) | 1.389 |
C(6) C(1) | 1.409 |
C(7)- C(6) | 1.455 |
C(8)- C(7) | 1.350 |
C(9)- C(8) | 1.489 |
N(10)- C(9) | 1.390 |
C(11)- N(10) | 1.374 |
S(12)- C(8) | 1.782 |
O(13)- C(9) | 1.212 |
N(14)- C(11) | 1.284 |
C(15)- N(14) | 1.365 |
S (16)- C(15) | 1.768 |
C(17)- S(16) | 1.757 |
N(18)- C(17) | 1.304 |
N(19)- C(15) | 1.304 |
S(20)- C(17) | 1.758 |
C(21)- S(20) | 1.858 |
C(22)- C(21) | 1.504 |
C(23)- C(22) | 1.399 |
C(24)- C(23) | 1.393 |
C(25)- C(24) | 1.394 |
C(26)- C(25) | 1.394 |
C(27)- C(26) | 1.393 |
Bond Angles | |
C(3)- C(2)- C(1) | 120.500 |
C(4)- C(3)- C(2) | 119.666 |
C(5)- C(4)- C(3) | 119.948 |
C(6)- C(1)- C(2) | 120.686 |
C(7)- C(6)- C(1) | 124.751 |
C(8)- C(7)- C(6) | 132.057 |
C(9)- C(8)- C(7) | 119.795 |
N(10)- C(9)- C(8) | 108.861 |
C(11)- N(10)- C(9) | 118.997 |
S(12)- C(8)- C(7) | 129.305 |
O(13)- C(9)- C(8) | 127.355 |
N(14)- C(11)- N(10) | 120.475 |
C(15)- N(14)- C(11) | 121.821 |
S (16)- C(15)- N(14) | 119.183 |
C(17)- S(16)- C(15) | 85.844 |
N(18)- C(17)- S(16) | 114.093 |
N(19)- C(15)- N(14) | 127.560 |
S(20)- C(17)- S(16) | 121.011 |
C(21)- S(20)- C(17) | 99.780 |
C(22)- C(21)- S(20) | 108.907 |
C(23)- C(22)- C(21) | 120.589 |
C(24)- C(23)- C(22) | 120.637 |
C(25)- C(24)- C(23) | 120.090 |
C(26)- C(25)- C(24) | 119.724 |
Mulliken atomic charges
The Mulliken atomic charge analysis of (Z)-5-((Z)-Benzylidene)-2-((5-(benzylthio)-1,3,4-thiadiazole-2-yl)imino)thiazolidine-4-one (1a) in the gas phase reveals significant variations in electron density across the molecule, which are crucial for understanding its reactivity. The carbon atom 21C exhibits the highest electron density with a negative charge of –1.2873920, indicating its potential as a nucleophilic site capable of donating electrons. In contrast, 22C carries a positive charge of 1.1563720, indicating it is the most electron-deficient and likely to act as an electrophile. Atoms 6C and 9C both exhibit positive charges of 0.4513840 and 0.4915310, suggesting moderate electron deficiency. On the other hand, atoms 1C and 15C carry negative charges of –0.5571340 and –0.5473030, indicating higher electron density at these positions, making them nucleophilic as well. The molecule has areas with different charges, indicating that electron-rich regions (like 21C, 1C, and 15C) are likely to engage in nucleophilic attacks. On the other hand, electron-deficient atoms (such as 22C, 6C, and 9C) are more susceptible to nucleophilic attack. These differences influence the molecule’s chemical interactions and potential reaction pathways. This detailed charge distribution is crucial for predicting the compound’s behavior in chemical reactions and interactions with other molecules (Figure 3).
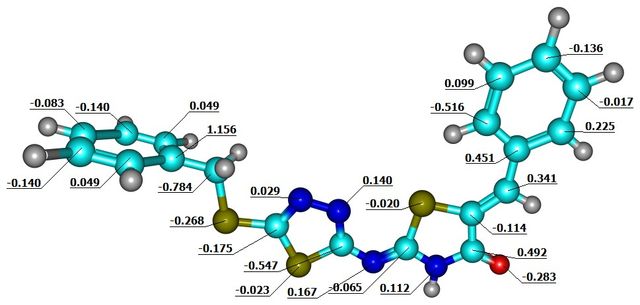
Global descriptors
The electronic properties of (Z)–5-((Z)-Benzylidene)–2-((5-(benzylthio)–1,3,4-thiadiazole–2-yl)imino)thiazolidine–4-one (1a) has been analyzed in terms of frontier molecular orbital (FMO) energies. An important factor in evaluating the chemical reactivity of coordination compounds is the energy gap (Eg = ELUMO − EHOMO), which represents the difference between the energies of the highest occupied molecular orbital (EHOMO) and the lowest unoccupied molecular orbital (ELUMO). In this study, we have calculated the global reactivity indexes, such as HOMO, LUMO, energy gap (Eg), hardness (\(\eta\)), softness (S), chemical potential (\(\mu\)), and electrophilicity index (\(\omega\)) for the titled complexes.
\(\eta = (E_{LUMO} − E_{HOMO})/2\)
\(S = 1/2\eta\)
\(\mu = − (E_{HOMO} + E_{LUMO})/2\)
\(\omega = \mu^2/2\eta\)
The reactivity indices are important for understanding the stability of molecules. The electrophilicity index (\(\omega\)) indicates how effectively a system can stabilize itself with electrons from the surrounding environment. Higher values of electrophilicity contribute to enhanced stability, light fastness, and photostability of compounds.
Chemical potential indicates a molecule’s stability, with negative values suggesting resistance to spontaneous decomposition. Hardness measures resistance to electron distribution changes and relates to stability and aromaticity. Molecules with a large HOMO–LUMO gap are harder and less reactive, while those with a small gap are softer and more reactive. Softness is the inverse of hardness, and electrophilicity reflects a molecule’s ability to accept electrons and reactivity. The electrophilicity index is used to assess toxicity, reactivity, and biological activity in drug-receptor interactions, based on HOMO–LUMO energy values. The calculated values of the reactivity descriptors for the ligand and complexes are presented in Table 2.
The small negative chemical potential (–0.16468 eV) indicates their stability, suggesting that these compounds are sensitive to decomposition. The magnitude of chemical hardness (0.06372 eV), supported by the HOMO–LUMO gap, suggests their resistance to deformation, indicating that they are polarizable and sensitive to changes in their electron cloud. The large global softness value (15.69366 eV) points to the soft nature of these compounds. The electrophilicity index (\(\omega\) = 0.00086403 eV), which relates to chemical potential and hardness, suggests very low nucleophilicity (Table 2, Figure 4).
HOMO (ev) | LUMO (ev) | Band-Gap (ev) | Electronegativity (ev) | Chemical hardness(\(\eta\)) (ev) | Chemical softness(S) (ev) | Chemical potential(\(\mu\)) (ev) | Electrophilicity(\(\omega\))(ev) |
-0.10096 | -0.2284 | 0.12744 | 0.16468 | 0.06372 | 15.69366 | -0.16468 | 0.00086403 |
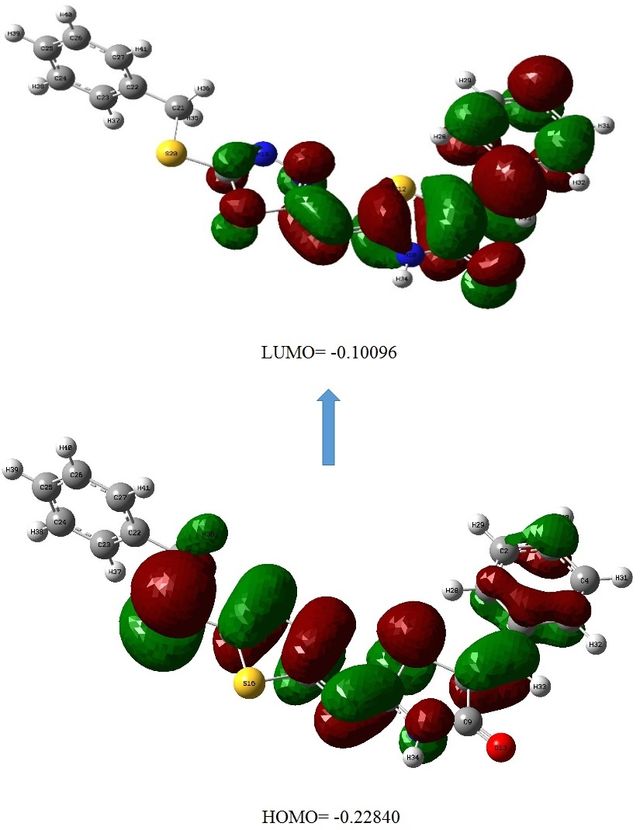
PASS Prediction and Bioactivity
The PASS online platform, recognized as one of the most reliable tools for predicting the bioactivity of potential compounds, was used to obtain prediction data (Pa > 0.7) [18, 21]. The PASS predictions are represented by Pa (the probability that a compound is active) and Pi (the probability that a compound is inactive). These probabilities range from 0.00 to 1.00, with Pa + Pi generally equaling 1, as these probabilities are calculated independently. Biological activities where Pa exceeds Pi are considered likely for a given compound. In this study, Pa > 0.7 was used to identify high-potential bioactivities (Table 3).
Entry | Compound | Mcl–1 antagonist | Antiviral (Rhinovirus) |
1a | ![]() | 0.805 | 0.732 |
1b | ![]() | 0.697 | 0.708 |
1c | ![]() | 0.719 | 0.713 |
Entry | Compound | Mcl–1 antagonist | Antiviral (Rhinovirus) |
1d | ![]() | 0.742 | 0.725 |
1e | ![]() | 0.711 | 0.716 |
1f | ![]() | 0.743 | 0.715 |
Entry
Compound
Mcl–1 antagonist
Antiviral (Rhinovirus)
1g
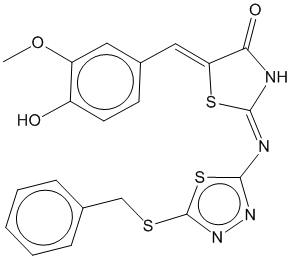
0.691
0.694
2a
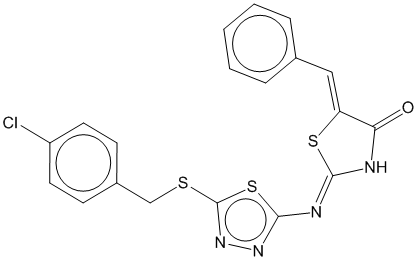
0.742
0.725
2b
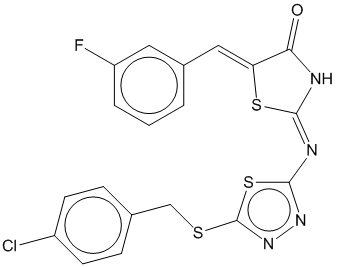
0.635
0.725
Entry | Compound | Mcl–1 antagonist | Antiviral (Rhinovirus) |
2c | ![]() | 0.665 | 0.705 |
2d | ![]() | 0.756 | 0.729k |
2e | ![]() | 0.708 | 0.661 |
Entry | Compound | Mcl–1 antagonist | Antiviral (Rhinovirus) |
2f | ![]() | 0.692 | 0.707 |
2g | ![]() | 0.647 | 0.688 |
3a | ![]() | 0.754 | 0.716 |
3b | ![]() | 0.649 | 0.687 |
Entry
Compound
Mcl–1 antagonist
Antiviral (Rhinovirus)
3c
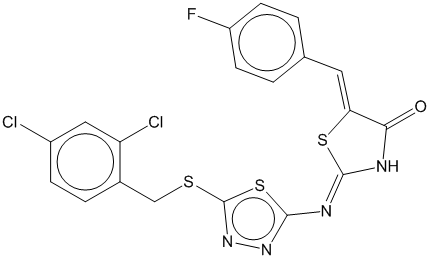
0.681
0.695
3d
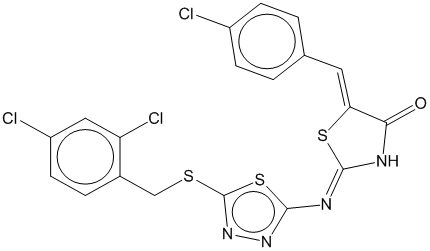
0.767
0.719
3e
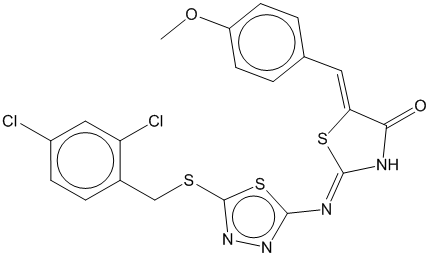
0.675
0.699
3f
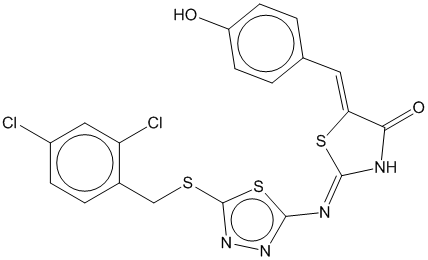
0.704
0.698
Entry
Compound
Mcl–1 antagonist
Antiviral (Rhinovirus)
3g
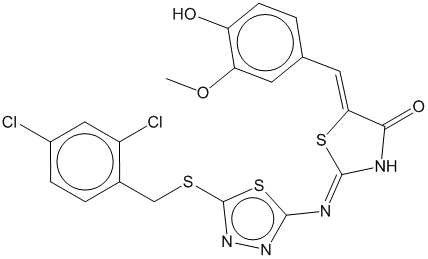
0.659
0.679
Docking
RdRP SARS-CoV–2 protein (PDB ID = 6M71 with resolution 2.90 Å) as protein targets were downloaded from Protein (http://www.rcsb.org). UCSF chimera has been used to perform the preparation of ligands and proteins. The preparation of protein target was carried out by removing water molecules, adding polar hydrogen atoms, and removing a natural ligand structure. Meanwhile, structure minimization of all ligands (1a-g, 2a-g. 3a-g, and Lopinavir, Nafamostat, Remdesivir as a standard ligand) was performed using UCSF Chimera before conducting the molecular docking analysis (Figure 5). The best binding affinities (more negative value) were selected from a set of 9 conformation poses after running docking. The compound showing the best hits was selected to visualize its molecular interaction. The visualization analysis was carried out using Discovery Studio software and depicted in 3D and 2D formats.
The molecular docking affinity scores of the ligands against the RdRP SARS-CoV–2 targets (6M71) ranged from –6.9 to –8.5 kcal/mol. Among these, compound 5c exhibited the strongest binding affinity with a score of –8.5 kcal/mol, followed closely by Remdesivir with a score of –8.4 kcal/mol. Visualization analysis was conducted using Discovery Studio software, presenting the selected interactions in both 3D and 2D formats. Binding affinities of –8 kcal/mol or lower are highlighted in grey in Table 4.
The docking positions of Remdesivir, Nafamostat, Lopinavir, and selected compounds with binding affinities of –8 kcal/mol or lower (1a, 1c, 1g, 2c, 2d, 2f, 3b, 3g) with the SARS-CoV–2 target were analyzed. The results show that Remdesivir, Nafamostat, and Lopinavir, along with compounds 2d (binding affinity = –8 kcal/mol), 2f (binding affinity = –8.1 kcal/mol), and 3b (binding affinity = –8.3 kcal/mol), occupy the same active site (Figure 6).
The interaction between the drug ligands and the SARS-CoV–2 target is primarily physical. This is evidenced by the binding affinity values in the Table 4, which range from –6.9 kcal/mol to –8.5 kcal/mol. These values suggest non-covalent interactions, such as hydrogen bonding, van der Waals forces, and electrostatic interactions, rather than the formation of covalent bonds typical of chemical interactions. Chemical interactions involve the sharing of electrons between atoms and usually result in irreversible binding with significantly higher affinities, often exceeding –10 kcal/mol. Since the binding affinities observed here are characteristic of non-covalent, reversible binding, it indicates that the drugs, such as Remdesivir and Nafamostat, are engaging in reversible, non-covalent interactions with the target, allowing them to dissociate and re-associate multiple times, which is typical for competitive inhibitors.
Entry | 6m71-Ligand | Binding Affinity | rmsd/ub |
1 | Remdesivir | –8.4 | 0 |
2 | Nafamostat | –7.9 | 0 |
3 | Lopinavir | –7.5 | 0 |
4 | 3g | –8.1 | 0 |
5 | 3f | –7.7 | 0 |
6 | 3e | –7 | 0 |
7 | 3d | –7.6 | 0 |
8 | 3c | –7.9 | 0 |
9 | 3b | –8.3 | 0 |
10 | 3a | –7.3 | 0 |
11 | 2g | –7.9 | 0 |
12 | 2f | –8.1 | 0 |
13 | 2e | –7.5 | 0 |
14 | 2d | –8 | 0 |
15 | 2c | –8.2 | 0 |
16 | 2b | –7.7 | 0 |
17 | 2a | –7.9 | 0 |
18 | 1g | –8.1 | 0 |
19 | 1f | –7.8 | 0 |
20 | 1e | –7.3 | 0 |
21 | 1d | –7.2 | 0 |
22 | 1c | –8.5 | 0 |
23 | 1b | –7.8 | 0 |
24 | 1a | –6.9 | 0 |
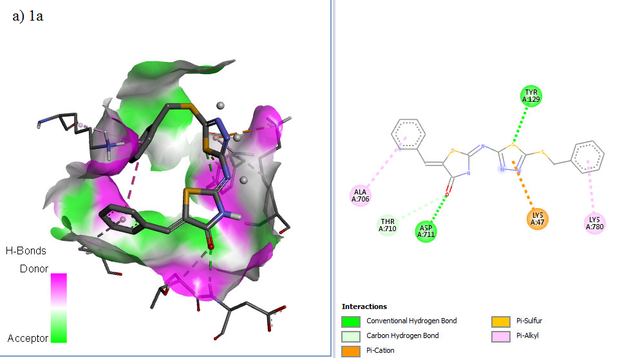
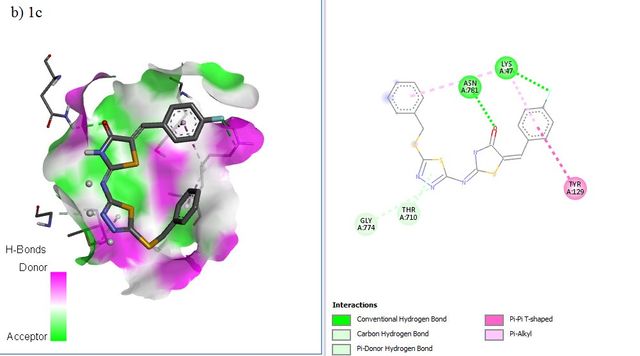
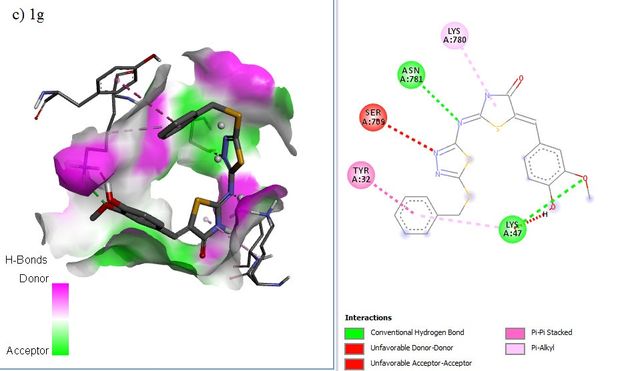
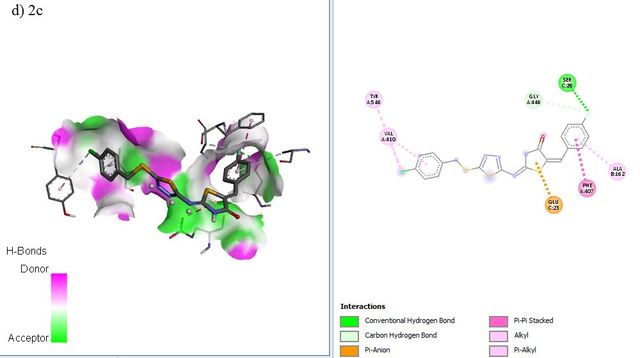
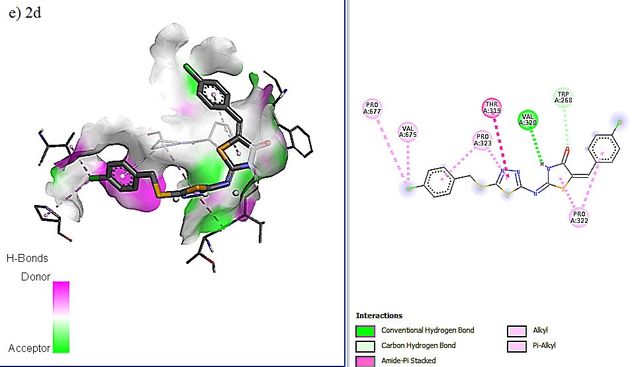
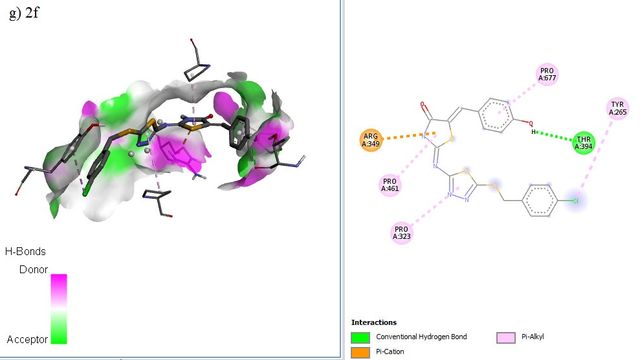
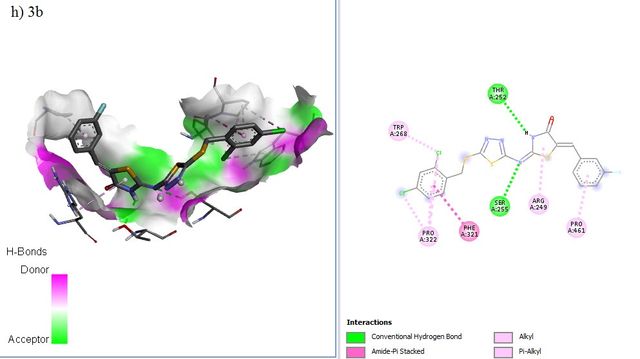
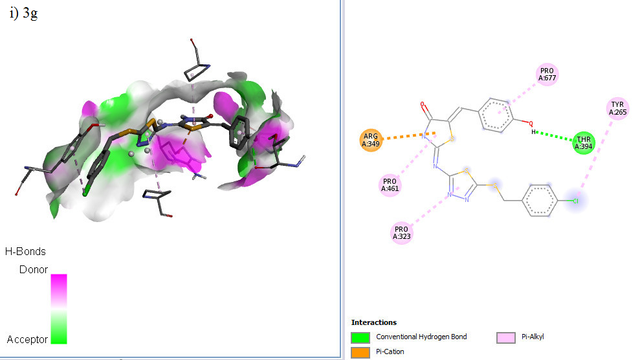
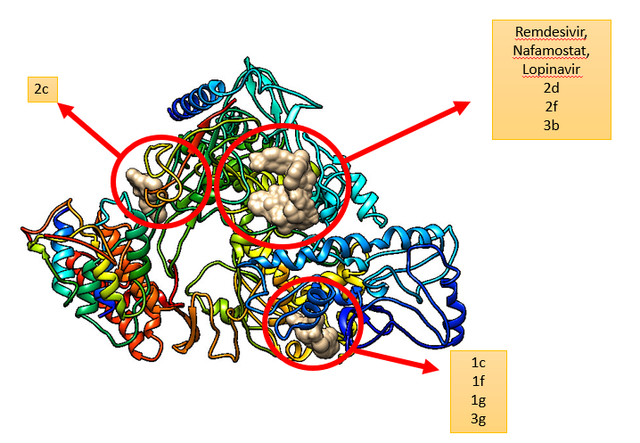
ADME Studies
It is important to evaluate the pharmacokinetics and toxicity of new therapeutic candidates [22-24]. In silico virtual screens and generative AI produce a large number of molecules, which need to be filtered to a manageable number for synthesis and experimental validation. One effective way to filter candidate compounds is by evaluating their Absorption, Distribution, Metabolism, Excretion, and Toxicity (ADMET) properties. ADMET-AI [19] is a user-friendly web interface that uses machine learning models to predict the ADMET properties of molecules. It is simple, fast, and accurate. For each input molecule, a radial plot is displayed to summarize five key ADMET properties in terms of their DrugBank percentile:
Blood-Brain Barrier Safe: The probability that the molecule does not cross the blood-brain barrier (i.e., 1 - Blood-Brain Barrier Penetration percentile).
hERG Safe: The probability that the molecule does not block the human ether-a-go-go (hERG) channel (i.e., 1 - hERG Blocking percentile).
Bioavailable: The probability that the molecule is orally bioavailable (i.e., Oral Bioavailability percentile).
Soluble: The aqueous solubility of the molecule (i.e., Aqueous Solubility percentile).
Non-Toxic: The probability that the molecule would not exhibit clinical toxicity (i.e., 1 - ClinTox percentile).
In this study, compounds 2d, 2f, and 3b (which occupy the same active site as standard ligands) were examined for their ADMET properties. The results indicate that they exhibit favorable druglike properties. Specifically, 2d and 3b have a lower total polar surface area (TPSA), leading to higher blood-brain-barrier (BBB) permeability compared to 2f (Table 5, Figure 7).
Lipinsky rule/compounds | 6d | 6f | 7b | Lipinski reference value |
HBD | 1 | 2 | 1 | <5 |
HBA | 7 | 8 | 7 | <10 |
MW Log P | 479.44 6.03 | 460.99 5.08 | 497.43 6.168 | <500 <5 |
TPSA | 67.24 | 87.47 | 67.24 | <140 |
Druglikeness | 0.36 | 0.41 | 0.34 | - |
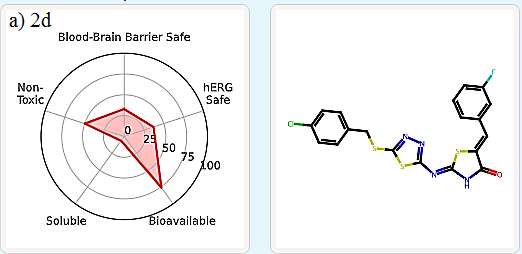
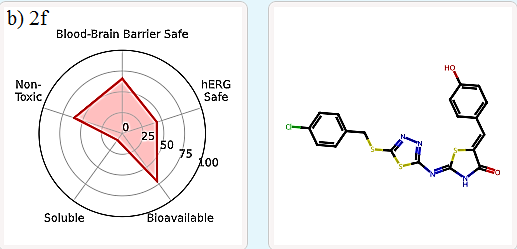
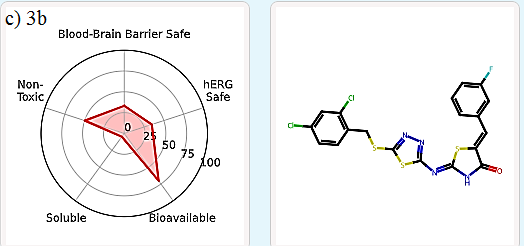
ADMET Optimization
Adsorption, distribution, metabolism, excretion, and toxicity (ADMET) qualities are critical for medication development and chemical risk evaluations of chemicals such as food additives, insecticides, and cosmetics. A major contributing cause to many drug development failures, unfavorable ADMET properties might raise safety issues for both human health and the environment. AdmetSAR3.0, an upgraded version of the popular ADMET assessment tool, offers advanced features for search, prediction, and optimization to enhance these assessments. For this purpose, ADMET of the compound 1a was optimized and evaluated, with the proposed suggestions (opt1, opt2, Figure 8) demonstrating improved bioavailability properties and enhanced values to 0.76 and 0.81 (Table 6) from 0.49 for non-optimized 1a.
Lipinsky rule/compounds | Opt1 | Opt2 | Lipinski reference value |
HBD | 1 | 2 | <5 |
HBA | 5 | 4 | <10 |
MW Log P | 426.55 3.57 | 409.5 2.43 | <500 <5 |
TPSA | 83.25 | 95.28 | <140 |
Druglikeness | 0.76 | 0.81 | - |
Finally, the results suggest that these compounds could be excellent candidates for further investigation. Therefore, conducting docking studies and ADME optimization before the synthesis and experimental phase is a beneficial approach to minimize costs and research time.
Conclusion
In response to the urgent need for new medications due to the COVID–19 pandemic, caused by the SARS-CoV–2 virus, modern drug discovery methods have been adopted. Bioinformatics has played a crucial role in speeding up the identification of drug targets and the development of effective treatments and vaccines. PASS predictions have pinpointed specific compounds with significant potential as potent Mcl–1 antagonists and antiviral agents. Using AutoDock Vina v1.1.2 in PyRx, 21 selected 1,3,4-thiadiazole and 1,3-thiazolidine–4-one binary heterocyclic molecules against the SARS-CoV–2 target (PDB ID: 6M71) has been studies. The calculated binding affinities, ranging from –6.9 to –8.5 kcal/mol, indicate that these compounds could be strong candidates for further investigation. Additionally, Density Functional Theory (DFT) calculations, which included molecular electrostatic potential (MEP) mapping, reactivity indices (such as electronegativity, electrophilic index, softness, and hardness), and frontier molecular orbitals (HOMO-LUMO), provided insights into the stability and reactivity of the selected compounds. ADME (absorption, distribution, metabolism, and excretion) assessments via ADMET-AI confirmed that these compounds exhibit drug-like properties suitable for medicinal chemistry. Further optimization of the ADMET properties using admetSAR 2.0 reinforces their potential in pharmaceutical applications. Ultimately, this study suggests that using bioinformatics tools and ADMET optimization is a valuable strategy for predicting compound efficacy before synthesis, potentially reducing costs and resources in drug development.
Acknowledgments
The authors gratefully acknowledge the financial support (grant no.1403) of this research by Shahid Chamran University of Ahvaz.
Statements and Declarations
Authors' Contributions
F. Heidarizadeh performed this study, Conceptualization, Funding acquisition, methodology, and article writing; S. Saadati performed this study and methodology, and E. Rostami performed this study, Conceptualization, Funding acquisition, methodology, and article writing.
Competing Interests
The authors declared no potential conflict of interest.
Ethics Approval
Not applicable.
Data Availability
All data generated or analyzed during this study are included in this published article.
Funding
The Shahid Chamran University of Ahvaz supported this work.
Authors’ Information
Fariba Heidarizadeh—Department of Chemistry, Shahid Chamran University of Ahvaz, Ahvaz, Iran.
Sadegh Saadati—Department of Chemistry, Shahid Chamran University of Ahvaz, Ahvaz, Iran.
Elham Rostami—Department of Chemistry, Shahid Chamran University of Ahvaz, Ahvaz, Iran.